Introduction
Advanced artificial intelligence models have made significant advancements, particularly in generative AI algorithms for imagery, text, and other data types, over the last several years. However, a fascinating new paper by data scientists both from Rice and Stanford University highlights a potential concern: feeding AI-generated content back into an AI model can lead to the erosion of their output quality.
In short, this self-consumption of AI-generated data creates an self-consuming loop, which the researchers term "Model Autophagy Disorder" (MAD).
How Does It Work?
A generative model could theoretically be consistently retrained on AI-generated material, simply by the prevalence of the content available on the internet. Natural language processing models aren't always aware of whether a particular piece of content was created by an AI model,
Over time, the quality of the model will degrade, simply from the natural process of individual factors being inadvertently overrepresented in the retrained data, fresh content being generated from that incorrectly trained data, and the neural network then being retrained yet again on its own misaligned content. The consequences of MAD are not yet well understood, but it raises important questions about the reliance on synthetic data in training next-generation AI models.
Understanding Model Autophagy Disorder (MAD)
In their research, the scientists found that without a sufficient influx of fresh real data in each generation of the autophagous loop, the quality (precision) or diversity (recall) of future generative models progressively decreases. The problem arises when machine learning models are repeatedly trained on synthetic data, leading to the disappearance of outlying and less-represented information present in the model's training data.
Eating Its Own
As a result, the model begins to rely on increasingly converging and less-varied data, causing it to deteriorate over time. The phrase 'garbage in, garbage out' is a common phrase in information technology circles for a reason!
MAD carries significant implications for the future of data science. If AI models are trained solely on synthetic content, their outputs start to exhibit cracks and limitations. In the study, the tested AI model showed signs of degradation after just five rounds of training with synthetic content. This finding raises concerns about the widespread use of scraping existing online data to train AI models, as well as the increasing reliance on synthetic content.
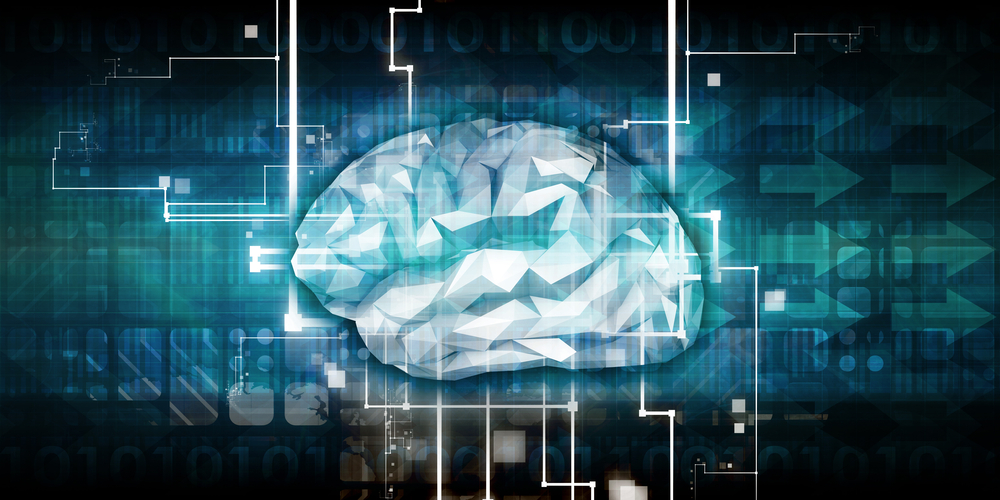
A Concerning Outlook
While the paper is yet to undergo peer review, it sheds light on the challenges faced by AI builders in an era where the internet is increasingly filled with AI-generated content. As AI becomes deeply intertwined with our online infrastructure, there is a growing need to ensure that training datasets contain sufficient fresh real data to prevent the degradation of AI model outputs.
The Price of Failure
Failure to address this issue may jeopardize the quality and structure of the open web. The exploration of Model Autophagy Disorder as a concept prompts us to consider the broader implications of AI's growing presence in our lives. As AI becomes increasingly intertwined with various aspects of society, including content creation, decision-making processes, and information dissemination, it is essential to ensure that AI remains a tool that serves the best interests of humanity.
Model Degradation
The degradation observed in AI models due to Model Autophagy Disorder (MAD) raises questions about the value of "fresh real data" in training AI systems. The researchers emphasize the importance of original human work as a data science opposed to AI-generated content. When AI models are trained repeatedly on synthetic content, the outlying and less-represented information at the outskirts of the training data gradually disappears. The model then relies on increasingly converging and less-varied data, leading to a decline in output quality and diversity.
'On its own supply'
This phenomenon carries significant implications for the future of AI training. Many AI models have been trained by scraping vast amounts of existing online data, with the belief that feeding more data leads to better models. However, the research findings indicate that without a continuous supply of fresh real data, AI models are prone to deterioration. This poses a challenge for AI builders who constantly seek more training material and face the risk of relying heavily on synthetic content.
Combatting the effect
The potential risks associated with the degradation of AI models highlight the importance of responsible AI development and deployment. It is crucial for AI developers, policymakers, and society as a whole to actively engage in ongoing discussions and establish guidelines that prioritize ethical considerations, fairness, transparency, and accountability.Model Autophagy Disorder prompts us to consider the broader implications of AI's growing presence in our lives.
As AI becomes increasingly intertwined with various aspects of society, including content creation, decision-making processes, and information dissemination, it is essential to ensure that AI remains a tool that serves the best interests of humanity.
AI's Role in Web Infrastructure and Content Creation
The current practice of scraping online data to train AI models has been the norm, with the belief that more data leads to deep learning and better models. However, the findings on Model Autophagy Disorder (MAD) call into question the consequences of training AI models solely on synthetic content. As AI-synthesized data becomes increasingly prevalent, it becomes challenging for AI companies to ensure that their training datasets remain free from synthetic content.
A part of the infrastructure
Major companies like Google and Microsoft have embedded AI in their search services, relying on AI-generated content to deliver relevant information to users. This integration of AI into web infrastructure raises important considerations. If AI models are compromised due to MAD, the outputs of these systems may be impacted, potentially leading to a decline in the quality and accuracy of search results, content recommendations, and other AI-powered services.
Implications for AI Training and Real-World Applications
The implications of Model Autophagy Disorder (MAD) go beyond theoretical concerns. Experiments with repeated generative image training on non-curated data indicate that degenerative artifacting begins to appear in as early as five iterations of the process.
The widespread use of AI models trained on synthetic content has tangible real-world consequences. Lawsuits against OpenAI and other organizations highlight the common practice of training AI models by scraping online data.
A growing reliance on AI, and what this means
As AI becomes embedded in various applications, including content generation and search services, the reliance on language processing of AI-synthesized data increases.
As more generative models are trained on AI-generated data, the risk of MAD intensifies. The popular LAION-5B dataset used to train text-to-image models, such as Stable Diffusion, contains synthetic images sampled from earlier generations of generative models. Even text sources that were once produced by humans are now increasingly generated by AI models without clear indications that they are synthetic.

A Growing Problem
The researchers warn that as the use of generative models continues to grow rapidly, the situation will only worsen. The prevalence of synthetic content on the internet makes it increasingly challenging for AI companies to ensure that their training datasets remain free from such content. This dilemma raises concerns about the quality, integrity, and structure of the open web, as the reliance on AI-synthesized data becomes more prominent.
mitigation strategies
Finding effective mitigation strategies becomes crucial. Adjusting model weights and developing techniques to maintain output quality are potential avenues for addressing the challenges posed by supervised learning by MAD. However, ongoing research and development are essential to fully understand and tackle this issue. Responsible AI development requires striking a delicate balance between leveraging the benefits of generative models and ensuring that the training datasets include a substantial amount of fresh real data.
Mitigation Strategies for Model Autophagy Disorder (MAD)
Addressing the challenges posed by Model Autophagy Disorder (MAD) requires the development of effective mitigation strategies. While the research on MAD is still in its early stages, there are potential approaches that can help alleviate the degradation of AI models over time.
One possible strategy is to adjust model weights during training to maintain output quality and diversity. By carefully fine-tuning the parameters of the model, it may be possible to mitigate the negative effects of the autophagous loop. This approach could involve striking a balance between synthetic data and fresh real data, ensuring that the model remains exposed to a variety of inputs.
Another avenue for mitigating MAD is to explore techniques that encourage the inclusion of fresh real data in AI training. This could involve the development of algorithms that prioritize the selection of diverse and representative human-generated content. By incorporating a continuous stream of fresh data, AI models can maintain their ability to produce high-quality outputs.
Collaboration Is Key
Ongoing research and collaboration between AI researchers, industry experts, and policymakers are essential to understand the intricacies of MAD and develop effective mitigation strategies. The responsible and ethical development of AI technology demands proactive efforts to address the challenges posed by the erosion of AI model quality and diversity.
The open web, which serves as a platform for diverse and original human-generated content, may face threats as AI-generated content proliferates. The prevalence of synthetic content can undermine the credibility and trustworthiness of online information. Striking a balance between AI-generated and human-generated content becomes crucial to preserve the quality, diversity, and integrity of the web.
The Importance of Human Input
The research findings on Model Autophagy Disorder (MAD) underscore the vital role of human input in AI systems. Without fresh real data contributed by humans, AI models suffer from degradation and limitations in their output quality and diversity. This suggests that machines and computer science alone cannot replace human creativity, critical thinking, and the ability to produce original and diverse content.
The limitations of AI systems without human input offer a glimmer of hope. It reaffirms the significance of human involvement and expertise in maintaining the integrity of information and ensuring the outputs of AI models remain reliable and relevant. Human creativity, nuanced understanding, and ethical decision-making are essential components that AI systems cannot replicate entirely.
In theory, anyway
The integration of AI and human collaboration is crucial to leverage the benefits of AI while mitigating the risks associated with Model Autophagy Disorder. Striking the right balance requires careful consideration, ensuring that human input remains a fundamental component in AI systems. By acknowledging the value of human intelligence and contribution, we can create a future where AI and human expertise coexist harmoniously, enriching our digital landscape and preserving the integrity of information on the web.
AI's Future and the Role of Human Expertise
The emergence of Model Autophagy Disorder (MAD) raises profound questions about the future of AI and the crucial role of human expertise in its development and deployment. The research findings highlight the limitations of AI systems without a continuous supply of fresh real data and the need for human input to ensure their integrity.
While AI has demonstrated remarkable advancements in various domains, it remains clear that AI cannot replace human creativity, critical thinking, and ethical decision-making. Human expertise is indispensable in curating, validating, and producing original content that reflects diverse perspectives and ensures the credibility of information.
Using AI responsibly
The challenges posed by MAD also highlight the importance of responsible AI practices. AI developers and organizations must prioritize transparency, accountability, and robust ethical frameworks when deploying AI systems. By integrating human expertise into the development and decision-making processes of artificial intelligence, we can create AI technologies that augment human capabilities, preserve the quality of information, and contribute positively to our digital ecosystem.
Conclusion
The discovery of Model Autophagy Disorder (MAD) highlights the challenges associated with training AI models on synthetic content. Feeding AI-generated data to an AI model without a continuous supply of fresh, naturally generated data can lead to a decline in output quality and diversity. The erosion of AI model performance raises concerns about the future of AI training and its impact on the web's integrity.
The implications of MAD extend beyond theoretical concerns. As AI becomes increasingly intertwined with web infrastructure, the reliance on AI-synthesized data poses risks to the quality and credibility of online information. Striking a balance between AI-generated and human-generated content becomes crucial to preserve the diversity and authenticity of the web.
Mitigation strategies, such as adjusting model weights and prioritizing fresh real data, are avenues for addressing the challenges of MAD. Ongoing research and collaboration among AI researchers, industry experts, and policymakers are essential to develop effective mitigation techniques and ensure responsible AI deployment.
Humans aren't outmoded... yet
The emergence of MAD emphasizes the significance of human intelligence and computer science expertise in the development and deployment of an AI model. Despite the significant progress natural language processing models and artificial neural networks have made in the past several years, human data analysis, creativity, and ethical decision-making still play vital roles in maintaining the integrity of information and ensuring reliable AI outputs. AI technologies should be designed to augment human capabilities rather than replace them entirely.
Big data companies wishing to lead in artificial intelligence sectors must regard it as crucial to prioritize responsible AI practices that uphold transparency, accountability, and ethical frameworks. Machine learning algorithms and AI techniques cannot fully replicate human behavior, and AI tools are only as good as the quality of their input.
A Collaborative Effort
Artificial intelligence technology should foster a collaboration between AI and human expertise rather than supplant it. Properly curated data can help a powerful AI model in solving complex problems, making accurate predictions and addressing real world problems. In the same way, classification problems and bad data can affect the way that AI models interpret basic concepts, by introducing classification based problems when unsupervised learning occurs.
One of the most important skills needed by a machine learning engineer may need in the coming artificial intelligence field may not be learning another programming language, or even how to operate a computer at all. Rather, an important skill in the developing economy may be to properly evaluate input and output variables for data consistency and veracity. Until a machine learning model learns the ability to recognize patterns created by self-reproducing datasets, MAD could cause your artificial intelligence solutions to go, simply put, mad.